Image Annotation Machine Learning: Unlocking Business Potential
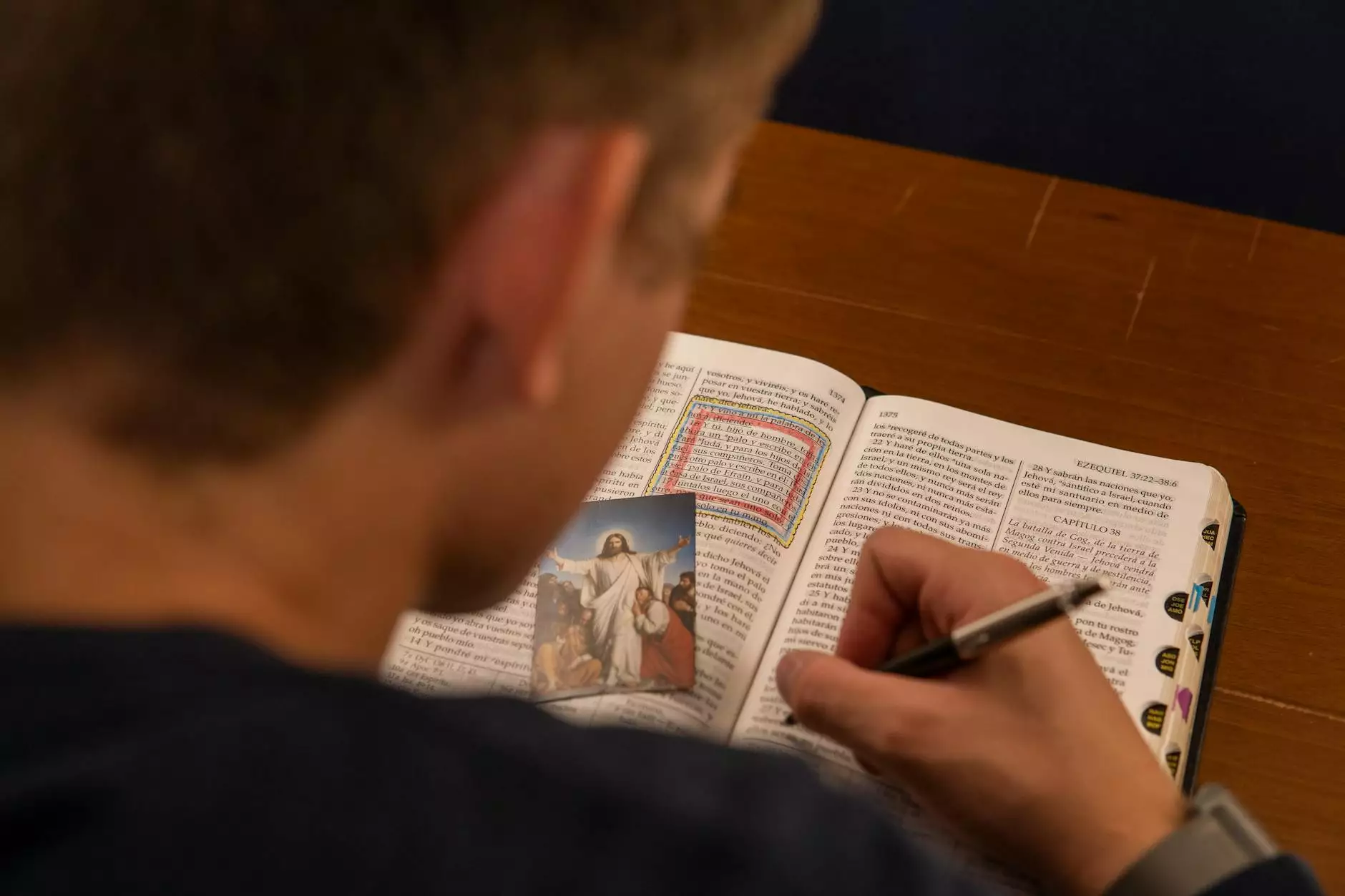
Introduction to Image Annotation and Machine Learning
In the ever-evolving landscape of technology, image annotation machine learning has emerged as a critical tool for businesses seeking to leverage the power of data. As organizations continue to collect extensive datasets, the ability to accurately annotate images is no longer a luxury; it is a necessity for driving business innovation and efficiency.
The Importance of Image Annotation in Machine Learning
Image annotation involves labeling images with relevant tags or metadata, enabling machine learning algorithms to understand and classify visual data. This process is essential for training models to perform tasks such as object detection, image classification, and image segmentation. Here are a few key points on why image annotation is vital:
- Enhances Data Quality: Properly annotated images ensure that the data fed into machine learning models is of high quality, leading to more accurate predictions.
- Boosts Model Performance: Models trained on well-annotated datasets exhibit improved performance in real-world applications.
- Facilitates Automation: By using annotated images, businesses can automate processes like quality assurance and defect detection.
Applications of Image Annotation in Business
Numerous industries are experiencing a revolution thanks to image annotation machine learning. Here are a few sectors where these innovations are making significant impacts:
1. E-commerce
In the e-commerce sector, product recognition and categorization are crucial. Annotated images allow machine learning algorithms to identify products quickly and accurately, enhancing the shopping experience. Image annotation helps in creating personalized recommendations, significantly increasing sales and customer satisfaction.
2. Healthcare
In healthcare, annotated imaging data is invaluable for training diagnostic models. These models assist in identifying anomalies in X-rays, MRIs, and other medical imaging techniques, improving patient outcomes. The role of image annotation in healthcare extends to effective interaction between physicians and AI systems, thus streamlining workflows.
3. Autonomous Vehicles
The autonomous vehicle industry relies heavily on image annotation for object detection. Vehicles must accurately detect pedestrians, road signs, and other obstacles to navigate safely. Through robust image annotation processes, machine learning models become more adept at making real-time decisions.
Understanding the Image Annotation Process
The image annotation machine learning process typically involves several stages:
- Data Collection: Gathering images from various sources, which can include in-house data, stock images, or web scraping.
- Choosing the Right Annotation Approach: Selecting whether to leverage bounding boxes, segmentation masks, key points, or other techniques based on the intended outcome.
- Annotation Execution: Utilizing tools and platforms to conduct the actual image annotation process, often employing both automated processes and human validation.
- Quality Assurance: Implementing checks to ensure that the annotation is accurate and meets the necessary standards for training.
- Integration with Machine Learning Frameworks: Feeding the annotated data into machine learning models for training and validation purposes.
Challenges in Image Annotation
Despite the benefits, businesses encounter several challenges in image annotation. Some of these challenges include:
- Scalability: As datasets grow, scaling annotation processes can become cumbersome and costly.
- Consistency: Ensuring that all annotations are consistent across different images and annotators can be difficult.
- Resource Demands: Image annotation often requires significant human resources, which can be a bottleneck for many organizations.
Best Practices for Effective Image Annotation
To ensure successful image annotation machine learning, businesses should adhere to several best practices:
- Utilize Robust Annotation Tools: Invest in high-quality annotation software that supports various image types and annotation styles.
- Establish Clear Guidelines: Provide annotators with comprehensive guidelines to ensure consistency and accuracy.
- Implement a Feedback Loop: Incorporate mechanisms for continuous feedback and improvement within the annotation team.
- Monitor and Evaluate: Regularly assess the quality of annotations and the performance of the machine learning models trained on this data.
The Future of Image Annotation in Machine Learning
As technology advances, the future of image annotation machine learning looks promising. Emerging technologies such as AI-driven annotation tools and crowd-sourced data collection are revolutionizing the way businesses annotate images. Increased automation and enhanced algorithms are set to streamline annotation processes, ensuring that businesses can keep up with growing data demands.
Conclusion
In conclusion, image annotation machine learning is a game changer for businesses across various sectors. By improving data quality, accelerating product development, and increasing operational efficiency, the strategic use of image annotation can drive significant business growth. As organizations continue to adapt and evolve, embracing these powerful technologies will be essential to maintaining a competitive edge in the market.
Call to Action
If you are looking to enhance your business capabilities through advanced image annotation and machine learning, consider partnering with experts in the field. At keymakr.com, we offer cutting-edge solutions tailored to meet the unique needs of your organization. Transform your data into actionable insights today!