Automatic Image Annotation: Transforming the Data Annotation Landscape
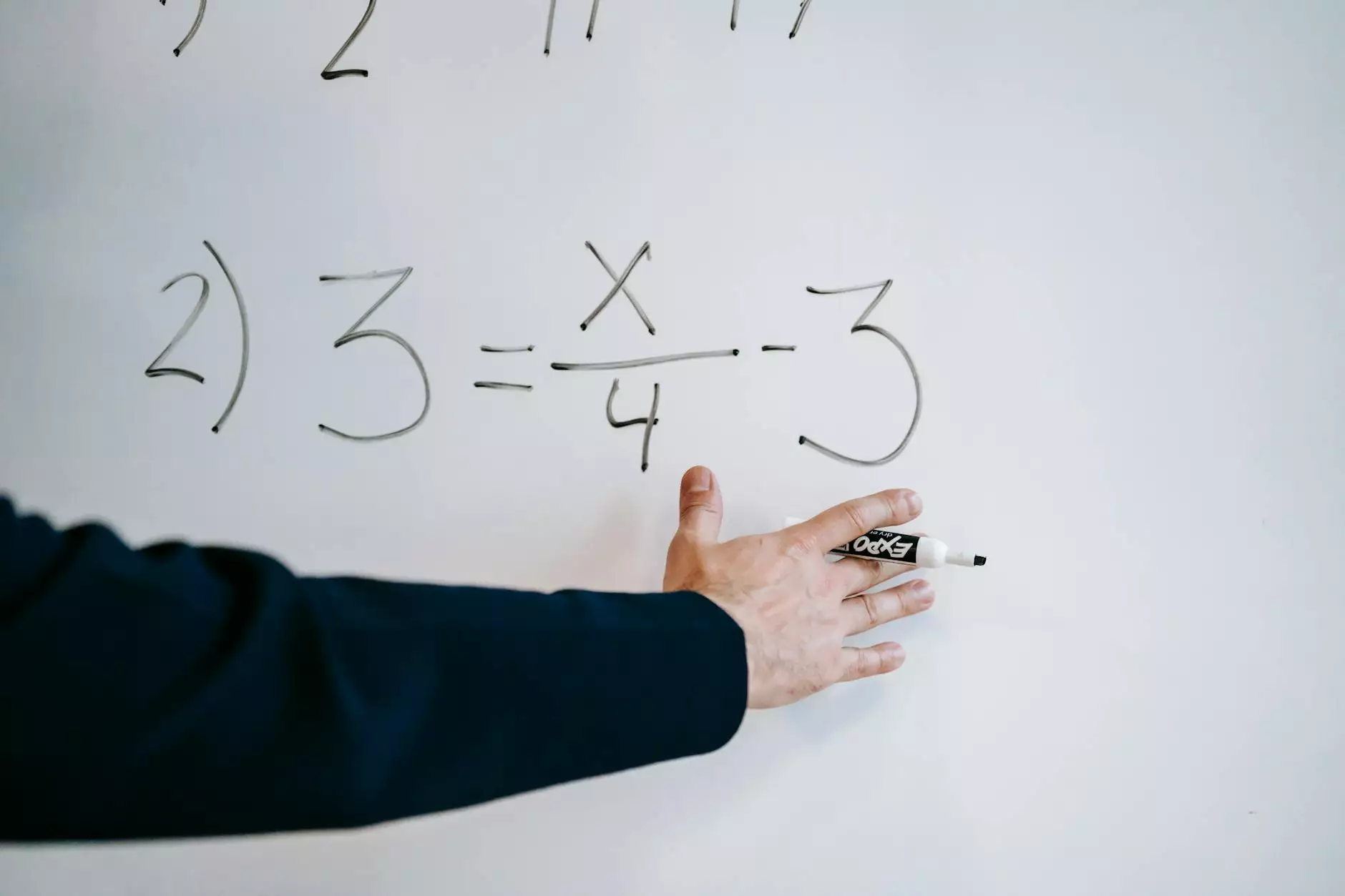
Automatic image annotation is a groundbreaking advancement in the realm of data annotation tools, offering significant advantages for companies that require vast amounts of annotated data for machine learning applications. This detailed article delves deep into the benefits and workings of automatic image annotation, showcasing why it is a game-changer in data annotation platforms.
Understanding Automatic Image Annotation
Automatic image annotation refers to the process of automatically labeling images using advanced algorithms and machine learning techniques. This technology relies on models trained on large datasets that allow for consistent and rapid annotation of images. As the demand for annotated data continues to surge, automatic image annotation stands out by providing a more efficient, scalable, and cost-effective solution.
The Importance of Data Annotation
Data annotation is the backbone of machine learning. It involves refining raw data, such as images, to teach machines to recognize patterns and make intelligent decisions. Here are a few functions that highlight its importance:
- Training Machine Learning Models: Accurate annotations improve the performance of AI models.
- Enhancing Computer Vision: Proper labeling helps systems interpret visual data.
- Facilitating Advanced Applications: Annotated data is vital for industries like healthcare, automotive, and retail.
Key Benefits of Automatic Image Annotation
1. Speed and Efficiency
One of the most significant advantages of automatic image annotation is the speed at which it operates. Traditional annotation methods can be labor-intensive and time-consuming. In contrast, automated systems can process thousands of images in a fraction of the time, allowing businesses to fast-track their projects and get data to market sooner.
2. Cost-Effectiveness
Manual annotation requires a dedicated workforce, leading to high operational costs. Implementing an automatic annotation system reduces these labor costs substantially. Businesses can allocate resources more judiciously, investing in core areas while benefiting from automated processes.
3. Scalability
As a business grows, so does its need for data. Automatic image annotation platforms scale seamlessly to handle increasing input without a significant drop in performance. This scalability ensures that organizations can continually enhance their machine learning models without the constraints associated with manual annotation.
4. Consistency and Quality
Machine learning algorithms used in automatic annotation are designed to maintain high levels of consistency across datasets. This ensures that the data is uniformly labeled, which is crucial for training reliable models. Human error is virtually eliminated, resulting in higher quality data.
How Does Automatic Image Annotation Work?
The process of automatic image annotation involves several key steps:
- Data Collection: Gathering a large dataset of images that will be used for training the model.
- Preprocessing: Cleaning and organizing the images to ensure they are ready for annotation.
- Model Training: Using labeled images to train the machine learning model to recognize and label new images accurately.
- Annotation: The trained model is then applied to new images to generate labels automatically.
- Validation: Finally, the annotations are checked for accuracy, ensuring the model is performing well.
Choosing the Right Data Annotation Tool
When considering automatic image annotation tools, businesses should evaluate several factors:
1. Compatibility
The tool must integrate seamlessly with existing systems and workflows. Evaluation of compatibility with current software and hardware is crucial.
2. Customization Options
Every business has unique needs. A good automatic image annotation tool offers customization options to cater to specific requirements and workflows.
3. Quality of Training Data
The effectiveness of an automatic annotation tool heavily relies on the quality of the training data used to develop the model. Tools that allow access to diverse and rich datasets will naturally yield better results.
4. Scalability
The chosen tool should have the capacity to grow with the business, managing larger datasets and increasing complexity as needed.
KeyLabs.ai: Revolutionizing Automatic Image Annotation
One standout platform in the realm of automatic image annotation is KeyLabs.ai. This state-of-the-art data annotation platform is designed with the latest AI technology, allowing for:
- Rapid Annotation Processes: Enjoy fast processing times with smart algorithms.
- User-friendly Interface: Simplified workflows enable better user experiences.
- High Accuracy: Machine learning models ensure highly accurate annotations.
- Seamless Integration: Effortlessly integrate with your existing AI infrastructures.
Best Practices for Using Automatic Image Annotation
To fully leverage the potential of automatic image annotation, consider the following best practices:
1. Regularly Update Training Data
Frequent updates to the training dataset can help improve model accuracy. Adding diverse examples reduces bias and improves generalization.
2. Validate Annotations
Even with automation, it’s essential to regularly validate results to ensure the model is producing quality annotations.
3. Monitor Performance
Consistently monitor the performance of the automatic annotation tool to identify areas for improvement. This could include adjusting the model or refining algorithms.
4. Collaborate with Experts
Engaging with data scientists and domain experts can provide invaluable insights into the effectiveness and application of the annotation results.
The Future of Automatic Image Annotation
The future of automatic image annotation looks promising as technology continues to advance. We are likely to see:
1. Enhanced AI Capabilities
With ongoing advancements in AI and machine learning, automatic image annotation will become even more sophisticated, potentially providing real-time annotative capabilities.
2. Increased Adoption Across Industries
As the benefits become more widely recognized, more sectors will adopt these tools to streamline their workflows and enhance decision-making processes.
3. Integration with Other Technologies
We may see a convergence of automatic image annotation with other technologies such as augmented reality, which can enhance interactive applications.
Conclusion
In conclusion, automatic image annotation is transforming the landscape of data annotation by offering unparalleled speed, cost-effectiveness, and consistency. With platforms like KeyLabs.ai leading the charge, businesses can harness the power of AI to enhance their operational efficiency and improve machine learning models. Adopting best practices and embracing these technologies will ensure that organizations are well-equipped to navigate the evolving data-driven landscape.
Investing in automatic image annotation is not just a trend; it is a necessary step for organizations aiming to stay competitive in an increasingly data-centric world.