The Importance of Bounding Boxes in Data Annotation
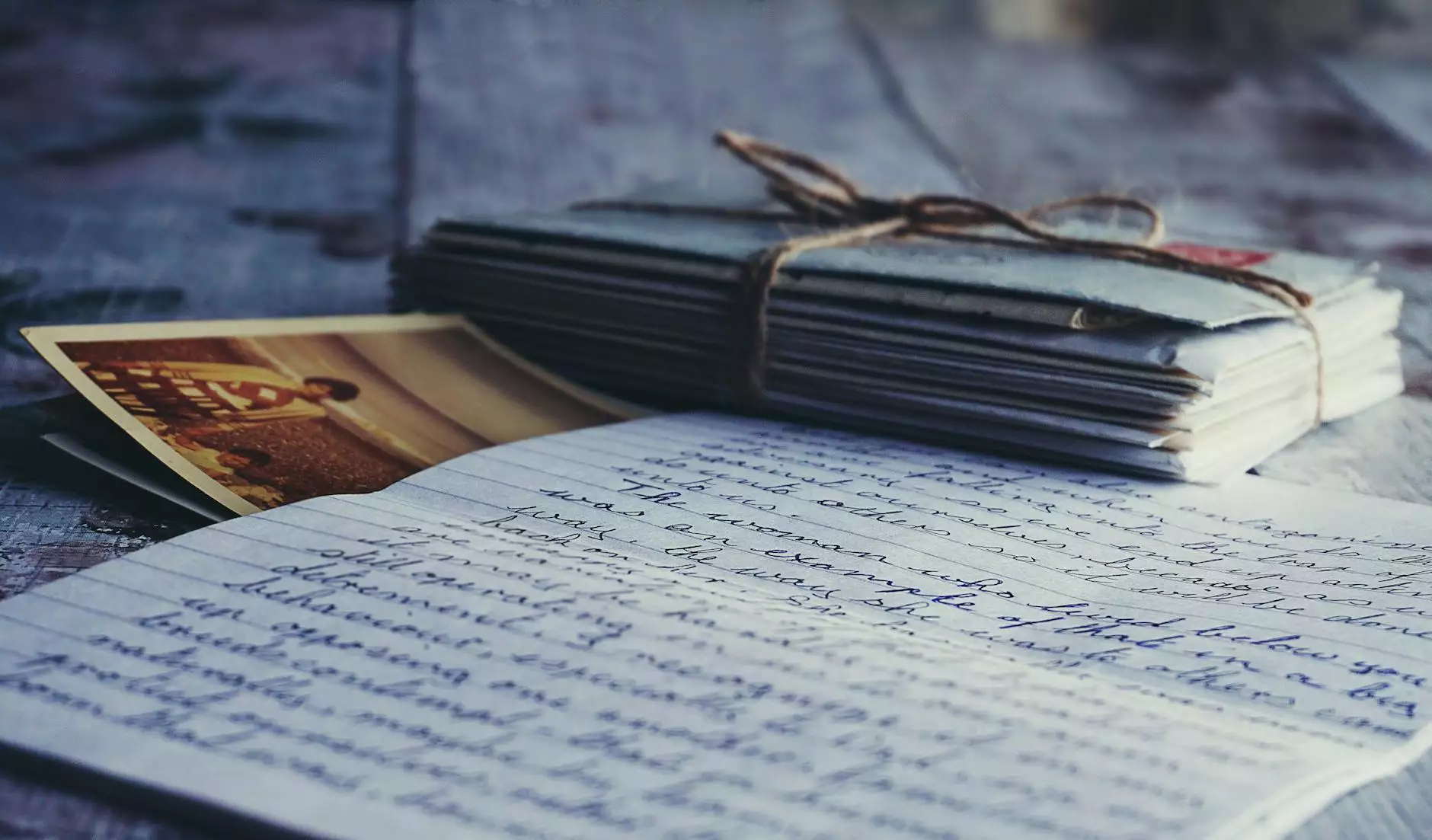
In the ever-evolving landscape of artificial intelligence (AI) and machine learning (ML), bouding boxes play a vital role in data annotation, particularly when extracting features from images and videos. At Keylabs.ai, we understand that efficient data annotation is the backbone of successful AI systems, enabling seamless training processes and ensuring high levels of accuracy in end results.
Understanding Bounding Boxes
To appreciate the significance of bounding boxes in data annotation, it's essential to define what this term means in the context of computer vision and graphics. A bounding box is a rectangular frame that encloses an object within an image. It is defined by its coordinates — typically the coordinates of the top-left corner, along with its width and height.
Why Use Bounding Boxes?
Bounding boxes serve several crucial purposes in the field of machine learning and computer vision:
- Object Detection: They help to localize objects within an image, which is essential for training models that can recognize various items in real-time.
- Data Management:Bounding boxes simplify the process of data annotation by providing a clear area of focus, making it easier for annotators to identify and label objects accurately.
- Performance Metrics: By utilizing bounding boxes, practitioners can determine the precision and recall of their models, allowing for better performance assessment.
The Process of Data Annotation Using Bounding Boxes
Data annotation involving bounding boxes typically follows these structured steps:
1. Image Selection
The first step is selecting and uploading the images that require annotation. Depending on the project, this can involve thousands or even millions of images.
2. Bounding Box Placement
Annotators use specialized software to draw rectangular boxes around the objects of interest in the images. This step requires attention to detail to ensure that the boxes closely match the shape and size of the objects.
3. Labeling
Each bounding box is then labeled with the appropriate class or type of object it represents. This process is critical for model training, as the model learns to recognize patterns based on these labels.
4. Review and Quality Assurance
To ensure high-quality annotations, a review process is implemented. This step may involve multiple annotators checking each other's work to confirm accuracy.
5. Exporting Data
After verification, the annotated data is exported in a format suitable for machine learning training, such as a JSON or XML file containing the bounding box coordinates along with their respective labels.
Benefits of Using Bounding Boxes in Data Annotation
The integration of bounding boxes into data annotation processes offers numerous advantages:
Enhanced Accuracy
Bounding boxes provide a clear, defined area that annotators can focus on, increasing the overall accuracy of the data. This precision is crucial for applications in fields like autonomous vehicles, security systems, and robotics.
Scalability
With effective annotation tools, the bounding box method allows for scaling up projects without compromising quality. As businesses increase their data needs, the ability to annotate large datasets becomes imperative.
Improved Training Data Quality
High-quality training data is paramount to developing robust AI models. With bounding box annotations, the training data can be curated to ensure optimal conditions for AI learning.
Keylabs.ai: Your Partner in Data Annotation
At Keylabs.ai, we pride ourselves on providing cutting-edge data annotation tools and platforms that simplify the use of bounding boxes for annotating complex datasets. Our solutions offer:
1. User-Friendly Interface
We offer intuitive software that makes the drawing and labeling of bounding boxes an effortless task, even for those new to data annotation.
2. Collaborative Features
Our platform supports team collaboration, allowing multiple users to work on data annotation projects simultaneously, streamlining the entire process.
3. Quality Control Measures
With integrated review systems and quality checks, we ensure that your annotated data meets the highest standards before it's exported for model training.
Challenges in Bounding Box Annotation
Despite its advantages, employing bounding boxes for data annotation comes with its challenges:
1. Ambiguity in Object Boundaries
Defining clear boundaries can sometimes be subjective, especially with overlapping objects or objects with irregular shapes.
2. Time-Consuming Processes
The manual process of drawing and labeling bounding boxes can become labor-intensive, particularly when dealing with large datasets.
3. Model Dependence
Models trained on poorly annotated datasets may not perform well in real-world applications. Hence, a rigorous annotation process is a must.
Alternatives to Bounding Boxes
Other data annotation methods exist that may serve different use cases:
- Polygonal Annotations: These allow for more complex shapes, especially useful for images with non-rectangular objects.
- Semantic Segmentation: In this method, every pixel is classified into a category, which can yield more detailed information than bounding boxes.
- Point Annotations: This technique is particularly beneficial for detecting specific objects or point features within images.
Future Trends in Bounding Box Annotation
The world of data annotation is rapidly evolving, and with it, the future of bounding boxes looks promising. Key trends include:
1. Automation and AI-Assisted Annotation
With advancements in AI, we can expect automated tools that can assist or even fully carry out the annotation process, reducing time and improving efficiency.
2. Integration with Deep Learning Models
As deep learning algorithms become more advanced, they will increasingly incorporate methods to fine-tune bounding box predictions and optimize model accuracy.
3. Real-Time Annotation
Future tools may allow for real-time annotations during video processing or live feeds, opening new applications in surveillance, autonomous navigation, and more.
Conclusion
Bounding boxes are an indispensable tool in the realm of data annotation, facilitating the training of advanced AI and ML models. By leveraging bounding boxes, companies can enhance their data quality, leading to superior outcomes in various applications, from self-driving cars to security systems. At Keylabs.ai, we are committed to providing state-of-the-art data annotation platforms that empower businesses to harness the full potential of their datasets. With our user-friendly interface and robust features, we make the annotation process efficient and accurate.
Investing in reliable data annotation with a focus on bounding boxes will undoubtedly future-proof your business in the competitive landscape of AI applications. Join us in revolutionizing how we annotate data and unlock the power of your data with Keylabs.ai.